[custom_breadcrumb]
DQE Unify vs Plauti Duplicate check
While Salesforce’s native deduplication tools are somewhat limited, there are excellent third-party applications available to help address and prevent duplicate entries. Two such tools are DQE and Plauti, which companies use to tackle their data quality issues. However, these products approach the challenge of duplication in different ways.
For more data quality info: Subscribe to our newsletter on Linkedin
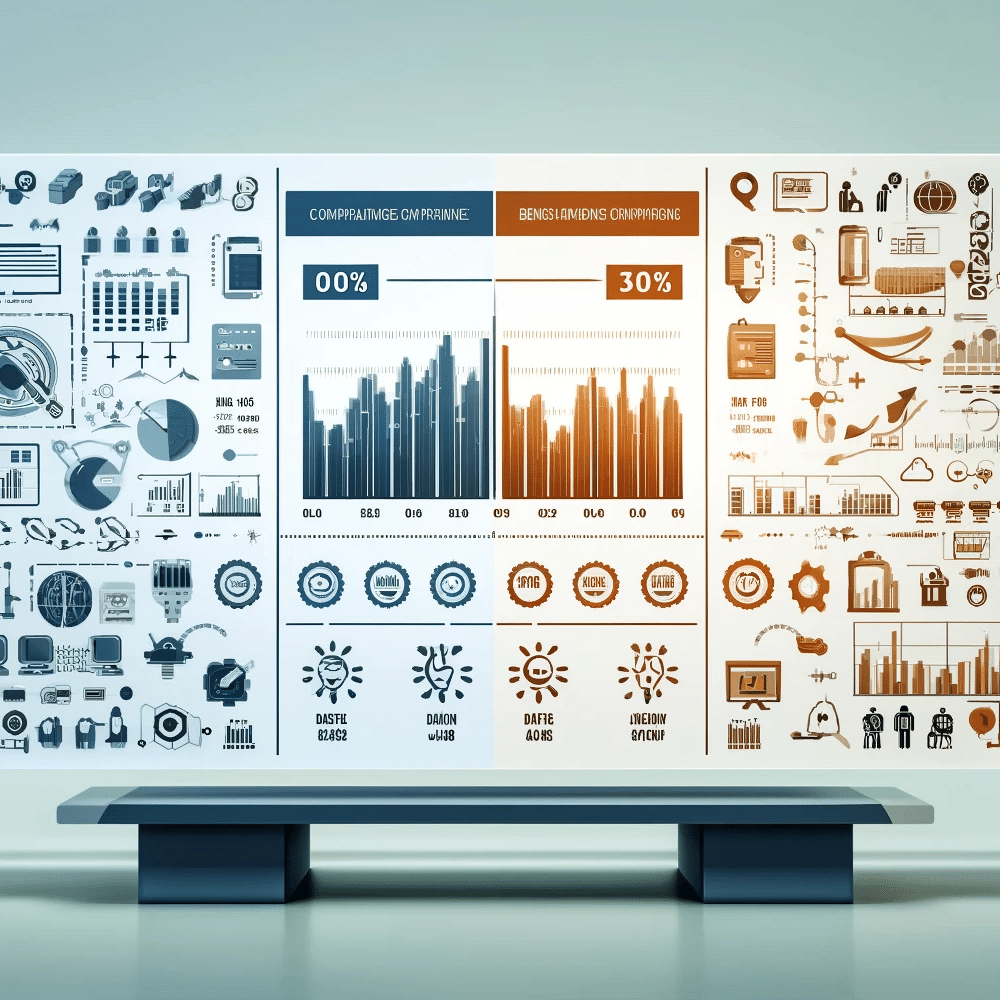
Both technologies have native interface in Salesforce and are easy to setup and use.
But, DQE goes even further by offering DAINA©, the first intelligent assistant that simplifies deduplication rules configuration.
You don’t need to set up the solution; simply communicate your data quality needs to our AI assistant, and it will take care of everything.
In its effort to seamlessly integrate with client IT environments, DQE has developed connectors for the leading cloud platforms on the market.
DQE vs Plauti: Ease of Use
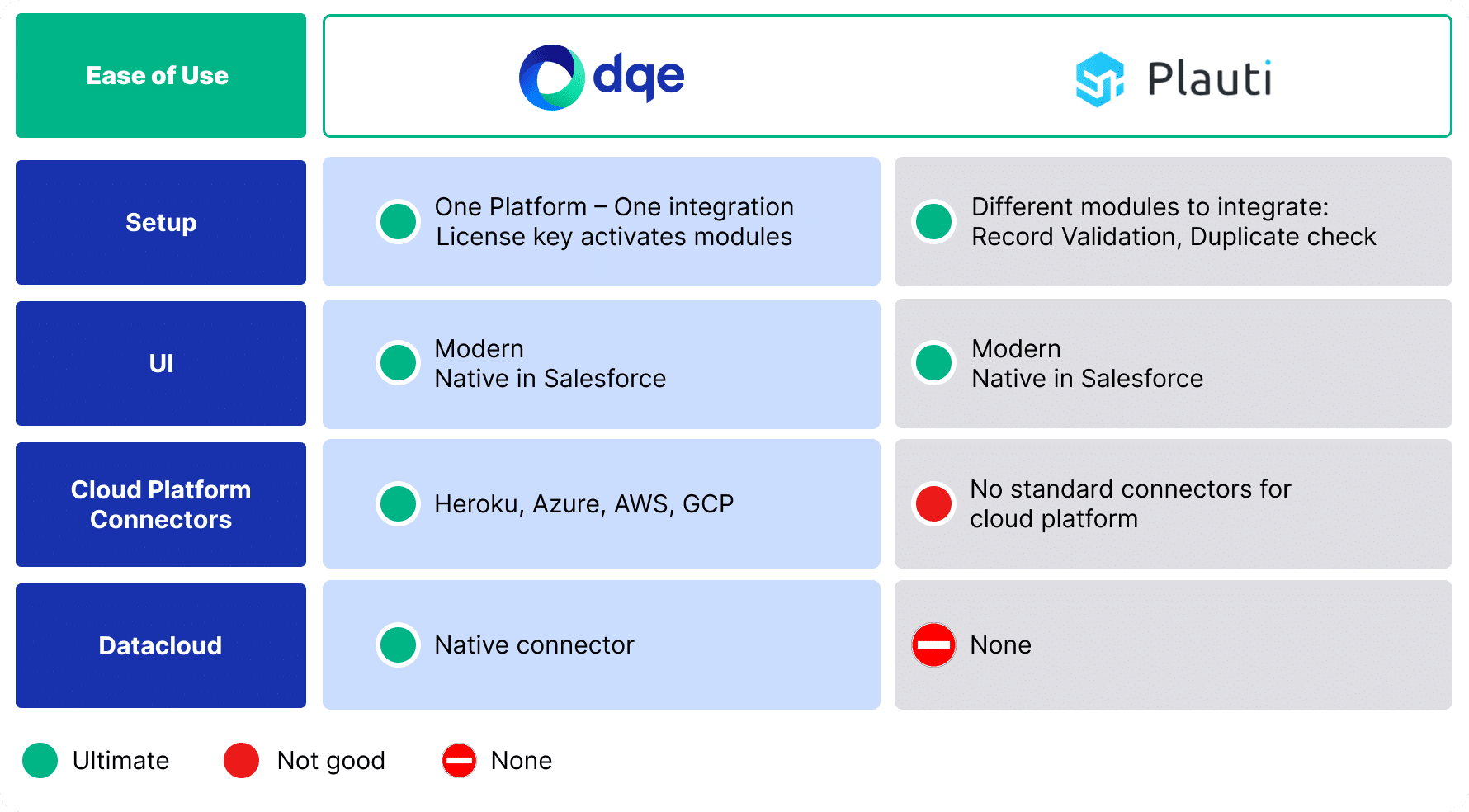
DQE vs Plauti: Match and Merge
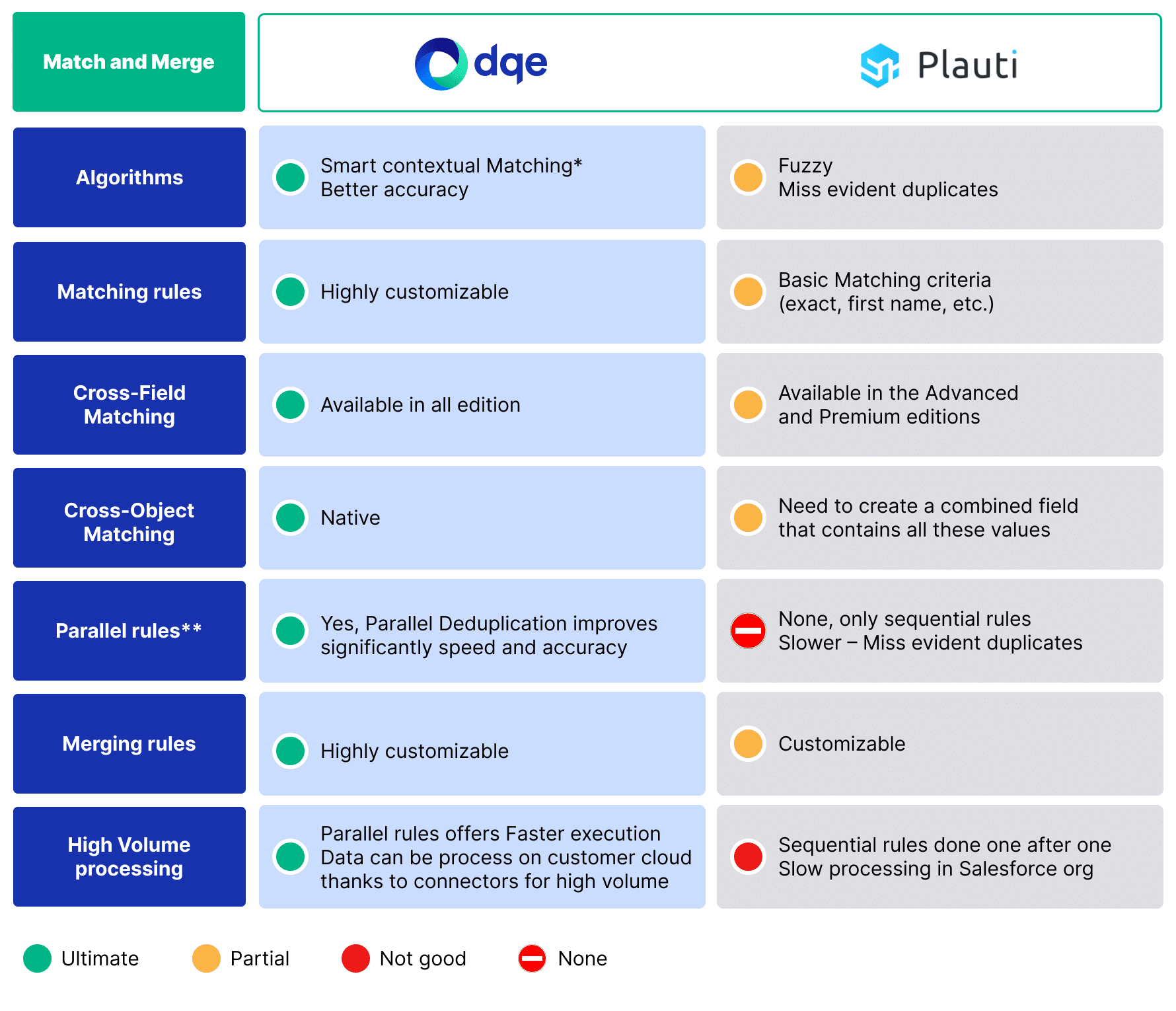
Conclusion
Plauti is suitable for simple deduplication needs. However, when it comes to providing expertise in deduplication, only DQE can deliver.
DQE can swiftly identify 10 to 20% more true duplicates compared to Plauti DC, thanks to our Smart Contextual matching and Parallel rules technology.
Deduplication Strategy
1- Fuzzy vs Smart Contextual Matching
Two Methods
- FUZZY MATCHING
Generic fuzzy-type algorithms use fuzzy matching techniques to identify similarities between data.
They do not take into account the specific context of the data.
- SMART CONTEXTUAL MATCHING
By integrating contextual intelligence into our matching process, SCM achieves a superior matching rate. This deep understanding ensures that even the most nuanced or complex matches are identified efficiently.
Comparison
- Smart contextual matching surpasses fuzzy matching due to its customization for specific data.
-
Fuzzy Matching may produce false positives, especially when dealing with ambiguous or noisy data. - SCM excel when deduplicating data with specific structures, like names and addresses.
- SCM considers factors such as variations in names (like initials or abbreviations), and spelling discrepancies.
=> Smart Contextual Matching improves significantly accuracy.
A Practical Example
Matching on First Name, Last Name, Mobile, Land Line, Email, Street, State, Country and Postal Code.
Fuzzy Matching:

Fuzzy Matching uses simple phonetic matching and Levenshtein distance algorithms, it did not match the third record because it could not match on any of the rules.
Smart Contextual Matching:

Smart Contextual Matching employs dynamic distance metrics and sophisticated pattern recognition to evaluate the similarity between data entries. It
matched all three records!
2- Sequential rules vs Parallel Rules
Two Methods
- SEQUENTIAL RULES
Sequential deduplication processes data one by one, comparing each piece with previously scanned data to identify duplicates.
It follows a linear and single-threaded approach to deduplication.
- PARALLEL RULES
Parallel deduplication divides data into segments and processes them concurrently across multiple threads.
It combines insights from each rule.
Comparison
- Parallel deduplication processes all rules simultaneously, saving time compared to sequential deduplication.
- Parallel deduplication offers a distinct advantage: it can still spot duplicates even if other rules find matches, significantly boosting precision compared to sequential methods.
- In sequential deduplication, evident duplicates are reduced due to its sequential process.
=> Parallel Deduplication improves significantly speed and accuracy.
A Practical Example
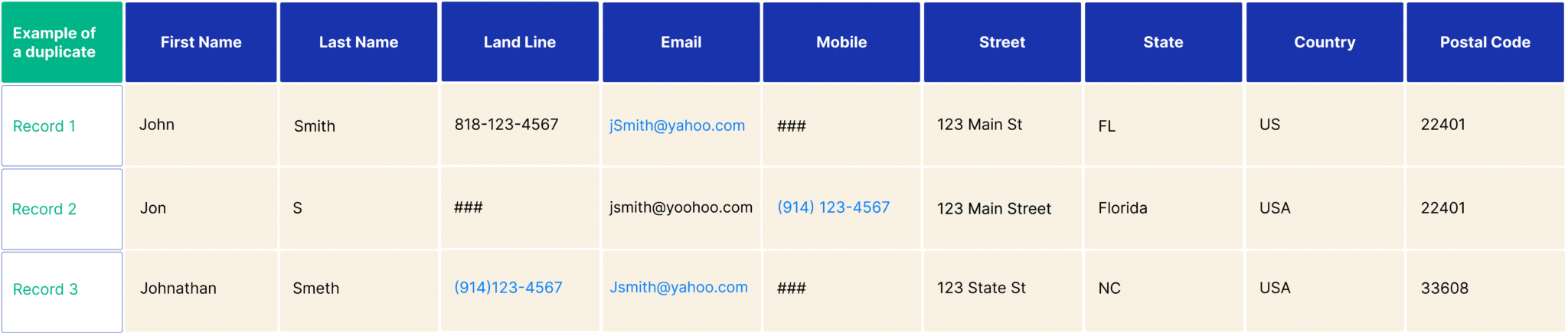
Result with Sequential Rules

In sequential deduplication with the initial rule of matching by email, the system initially grouped ‘John Smith‘ with ‘Johnathan Smeth‘ due to their shared email address (‘jSmith@yahoo.com‘ and ‘Jsmith@yahoo.com‘). After removing this duplicate, the system couldn’t link ‘John Smith’ with ‘Jon S’ as they didn’t share a common email or phone.
Result with a Parallel Rules

With parallel deduplication rules, multiple attributes of data entries are compared simultaneously. While one thread matches entries based on email addresses (e.g., ‘jSmith@yahoo.com‘ and ‘Jsmith@yahoo.com‘), another thread simultaneously identifies potential duplicates using other shared attributes like names ‘John Smith‘ and ‘Jon S‘ ,phone numbers ‘(914) 123-4567‘ or address ‘123 Main Street‘.
3- Merging with DataQ
In the example below, how can reliable contact data be differentiated from unreliable contact data before performing a merge?
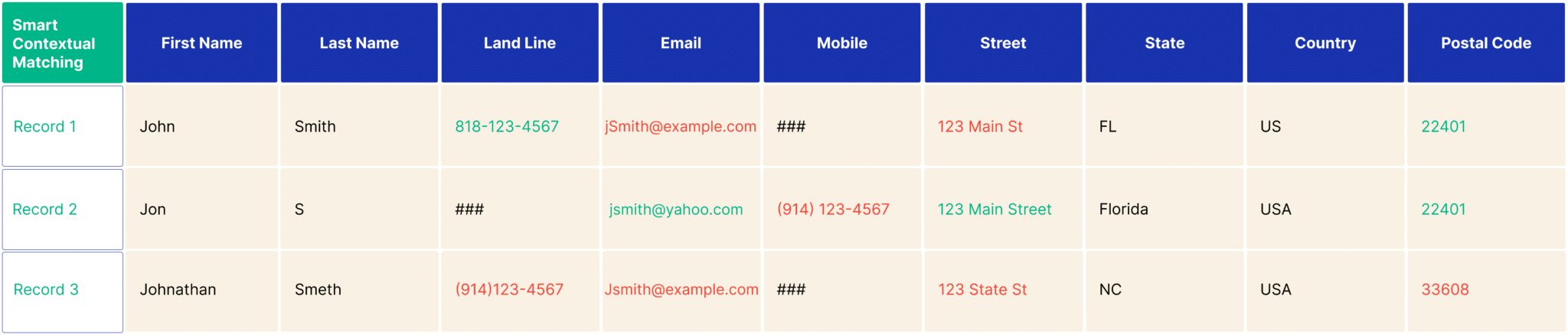
The same contact exists in triplicate, to perform intelligent data merging, DataQ verifies reachable contact data.
What DataQ brings to your merge

Single contact records enhanced with reliable contact data.
During deduplication, contact data (Postal Address, email, phone) is checked to determine its reachability.
This process enables the identification of the golden ID and facilitates Smart merging with reliable data by performing quality checks on contact details.
About DQE
Because data quality is essential to customer knowledge and the construction of a lasting relationship, since 2008, DQE has provided its clients with innovative and comprehensive solutions that facilitate the collection of reliable data.
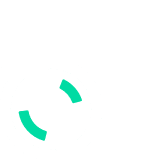
17
Years of
expertise
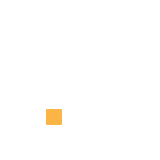
800
Clients in all
sectors
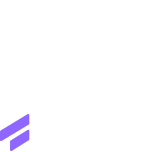
3Bn
Queries per
year
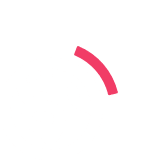