Data quality, vector of success for AI use cases
Data quality goes far beyond technical considerations. It lies at the heart of the success or failure of AI projects, affecting both the profitability and competitiveness of companies in a context of accelerated digital transformation.
For more data quality info: Subscribe to our newsletter on Linkedin
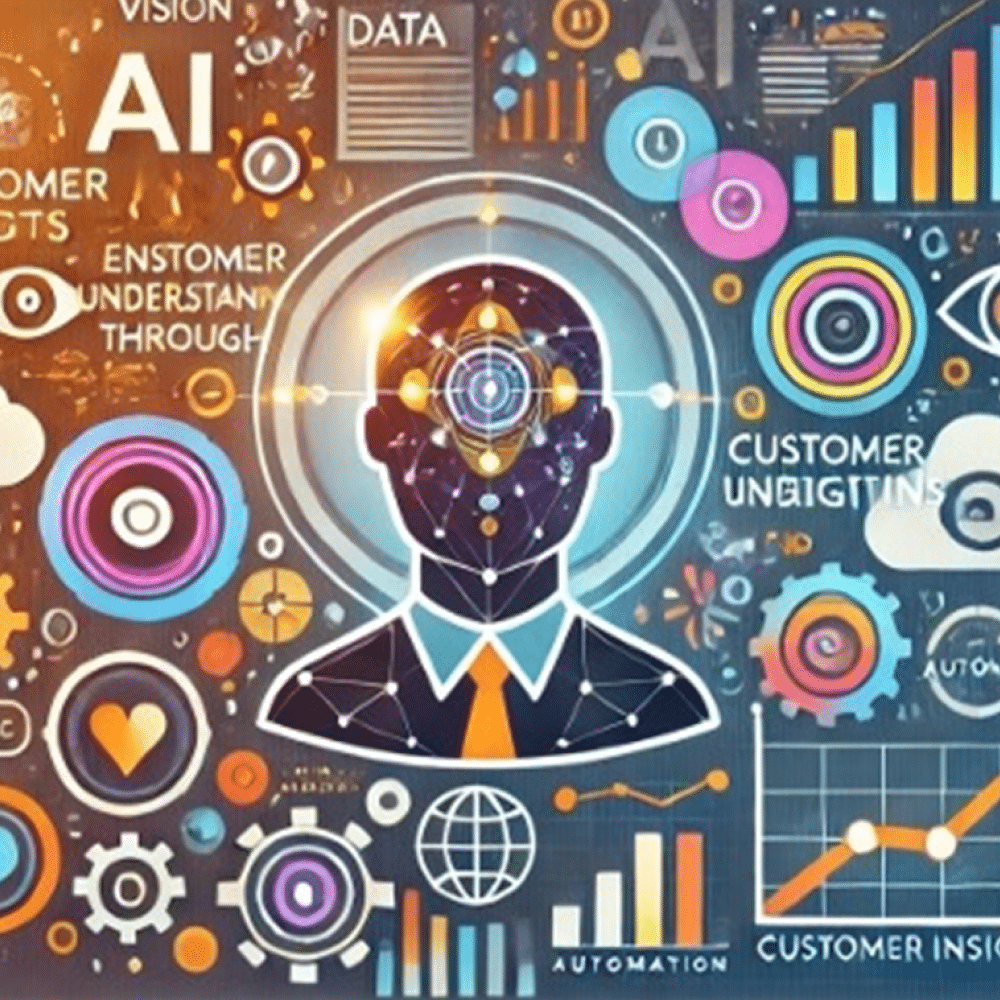
After a period of experimentation, generative AI is now entering a deployment phase in operational solutions. Advanced language models are now more widely available to mature technology teams, lowering barriers to entry.
However, AI must be trained with relevant and reliable data in order to deliver convincing results in its applications. That’s why, when customer data comes into play, the first thing to do is to qualify the elements of its foundation, i.e. customer contact data. What is at stake is the reliability of AI results, user confidence, and successful use cases. Let’s take a more detailed look.
AI and the goal of reliable data
Generative AI uses data at unprecedented scale and speed, drawing massively from vast repositories of data to respond to user queries. In doing so, it amplifies the importance of data reliability.
Generative AI’s need for vast amounts of data creates a major challenge for teams that manage data: the queries sent to the AI engine by users can neither be anticipated nor screened, making it impossible to know which datasets need to be prepared and cleaned to feed the AI’s responses. So, without qualified data, AI results may present errors and be unreliable, possibly making professionals that might use the technology doubtful of its applicability to their use cases.
In other words, AI accentuates the impact of poor data quality. When customer contact data contains errors or inaccuracies (duplicate email addresses, wrong names, incorrect mailing addresses, etc.), customer knowledge and the 360-degree view of customers are inadequate and AI magnifies these deficiencies. This means that, if the repository includes erroneous address data or duplicates coming from different email addresses from the same customer, it can’t bring up fine-grained information to assist sales reps according to their catchment area or the profiles of their customers.
AI without data quality: taking risks
Without qualified data, AI increases the risk of potentially biased answers, and users may be reluctant to adopt its use cases. A McKinsey study has confirmed this, with 70% of AI initiatives failing mainly due to poor quality data, which compromises the reliability of results.
Starting from the user’s query, up to the generation of the response, the process is exposed to numerous points of failure if the AI is working with unqualified data. For instance, AI will struggle to correctly associate customer data elements with each other if imperfections in contact data blur the lines defining contactability.
Moreover, once training data has been put into the engine, it becomes difficult to control which users have access to which data elements, creating new levels of uncertainty, as well as risks in terms of data protection. This includes unqualified customer contact data opening up entry points that hackers know how to exploit. Identity fraud is one example, where many scenarios involve the misuse of names and contact data, when this data has not been properly validated as true and exact with a high-performance data quality tool. This is the case, among others, for people entering a false address in order to escape payment reminders, or using SIM swapping to falsely validate a transaction.
Data quality is taking center stage today
“Garbage in, garbage out” is a logic that is particularly evident in AI projects. As such, 67% of business leaders are concerned about AI-related operational risks, mainly due to data governance issues and data quality*. In order to prevent generative AI results from undermining the adoption of AI use cases and user confidence, proactive approaches to data quality are now necessary. This is a significant development since data quality has long tended to be handled reactively, and wrongly so.
Another motivation for resolving data quality problems prior to processing is to justify AI investment, given the negative impact of processing poorly qualified data which degrades the profitability and sales value of projects. The cost of one data input error has been estimated to be between $10 and $100, linked to reputation, regulatory non-compliance, and missed opportunities if it is not corrected beforehand**. Data quality has therefore become a prerequisite for making AI projects profitable by using qualified and consistent data at the company level.
Last but not least, the massive volumes of data used in AI models increase the energy consumption of digital technology, and therefore its carbon footprint. Data cleansing helps to limit these impacts by removing unnecessary data from processing, lowering carbon emissions in the process, as demonstrated by DQE’s eco-calculator .
Also worth noting, according to a study carried out in 2023, companies that use advanced capabilities to better control their sustainable development data are 43% more likely to achieve better profitability than their competitors*. The stakes are high, and it is worth the effort to take these things into consideration.
At our last DQE Users’ Club meeting, many companies gave feedback on their experience with qualified data and the issues related to AI projects in their organizations. Here are some highlights of their experiences:
- Byredo: “Securing data is a critical issue for offering high-performance AI solutions to all services that are involved in the international expansion of our company.”
- SFR Business: “We need a true ‘augmented agent’ that is equipped with AI-based tools, in order to ensure optimal control of our data quality, all while managing the increasing complexity of our product offering and the types of customers we serve.”
- Belambra: “With the help of tools that are adapted to our work, our customer service teams can concentrate on tasks that generate value, such as increasing sales, while also reducing the time spent on tasks linked to after-sales service.”
- Rossel Advertising France: “Integrating data quality into the core of our digital transformation is not only an IT issue. It is a collective challenge that concerns the whole company, if we want our AI projects to succeed.”
The 3 areas of qualidied data that work to serve AI
Faced with the data quality challenges that are inherent to AI, companies need to stack the odds in favor of their new use cases by doing the groundwork on three levels:
- The human element: in an AI project, it’s important to bring together all the relevant business lines in the company to combine their operational visions and technical expertise. In terms of data quality, specifically concerning customer contact data, this collective approach helps the company to understand data use and the associated problems that need to be resolved before using AI. Reinforcing data culture for customer data also contributes to improving data quality by raising awareness of poor practices that have to be eliminated – manual imports of poorly qualified Excel files, untimely changes to the database, input of data that is incomplete or nonsensical, etc.
- Governance: AI means managing data and its quality on a large scale. This is why solid data governance is required to optimize the flows that feed AI. Data quality is an integral part of the equation.
- Technology: a data quality tool that is capable of collecting, reconciling, and unifying massive volumes of customer data in unified profiles that are accurate and up-to-date is proving essential to guaranteeing the reliability and effectiveness of AI models. In addition, the data quality tool must be able to curate vast volumes of data so that the AI takes in incoming qualified data without delay.
As such, the importance of data quality goes far beyond technical considerations. It lies at the heart of the success or failure of AI projects, affecting both the profitability and competitiveness of companies in a context of accelerated digital transformation.
*Source: DataTrails, October 2023
**Source: Data Driven: Profiting from Your Most Important Business Asset, Thomas C. Redman, 2008
**Source: Data Driven: Profiting from Your Most Important Business Asset, Thomas C. Redman, 2008
About DQE
Because data quality is essential to customer knowledge and the construction of a lasting relationship, since 2008, DQE has provided its clients with innovative and comprehensive solutions that facilitate the collection of reliable data.
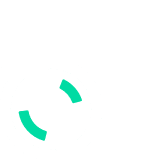
17
Years of
expertise
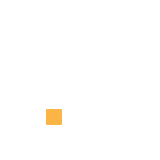
800
Clients in all
sectors
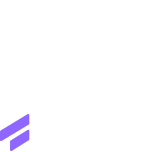
3Bn
Queries per
year
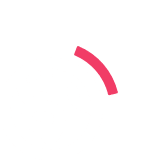