5 universal strategic challenges that can be solved by data quality
For more data quality info: Subscribe to our newsletter on Linkedin
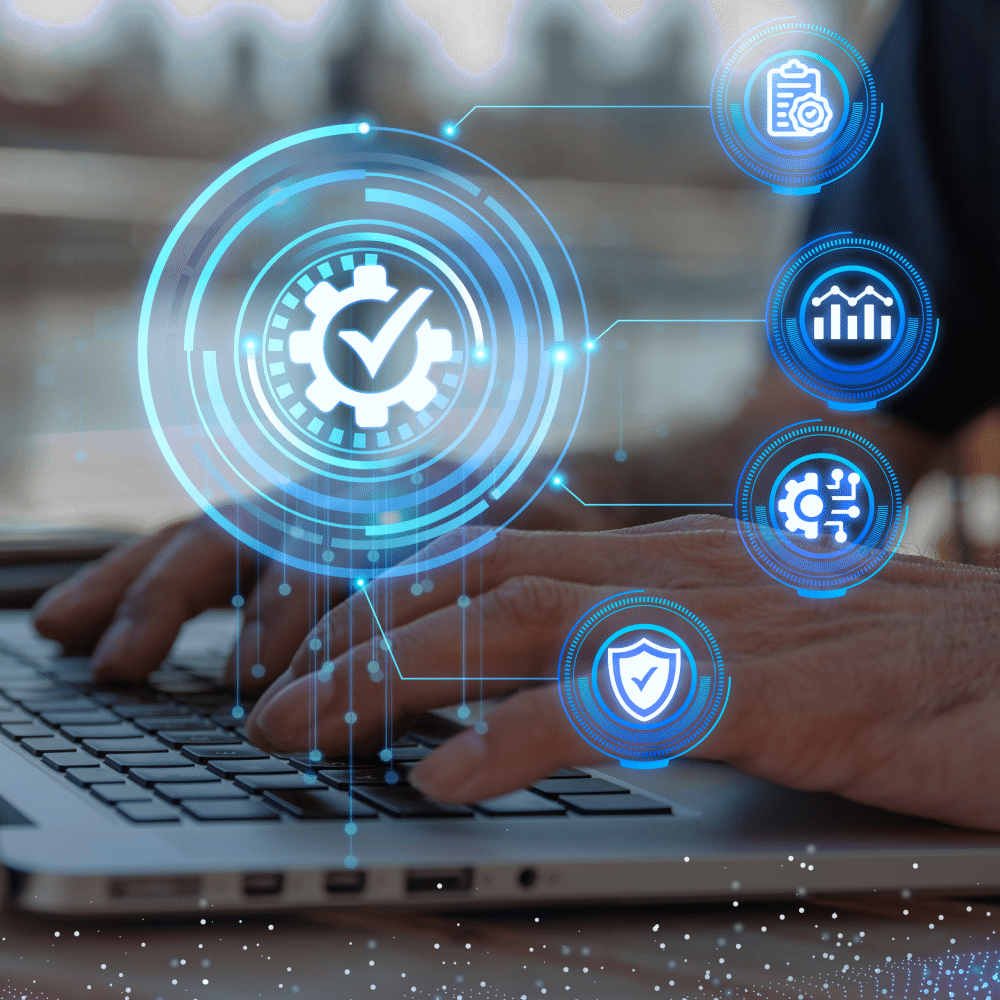
According to Kompass, a database loses an average of 30% of its value each year. This means that poor quality data has a real cost: amounting up to 20% of annual revenue according to Gartner, and 20% of productivity according to Kompass. To overcome these obstacles, businesses have to understand what role data quality plays in their performance challenges. They have everything to gain in that regard, especially in terms of customer relations, business development, cutting costs, compliance, and reputation
Surveys on data quality show multiple problems in companies that have not made efforts to cleanse their customer data. Awareness is growing, but 59% of brands still do not measure the quality of their data, according to Gartner. However, qualifying customer data helps avoid many problems:
1. Putting a stop to missing the target customers and prospects
Businesses very often report that exchanging with prospects and customers is a challenge for them. Several DQE clients confirm that contactability plays a role in their data quality approach. “We need to have a correct email address and a correct phone number for contacting customers via WhatsApp or text message, in order to create an exchange that brings customers back to the store later, creating repeat business,” explained Orianne Joannic, IT and CRM Project Manager at Byredo. Yet, without contact data that is verified and correct, the ROI on relational campaigns is diminished. The reason: too many messages never make it to their target, whether they be emails, text messages, or traditional mail. It should be noted: 30% of email addresses become invalid each year (HubSpot), and 48% of outgoing B2C calls fail due to invalid or blocked numbers (Gartner). Performance in terms of engagement of target customers drops, and unnecessary costs arise.
Missing a target can also be linked to an increased number of duplicate entries, which is responsible for the loss of a customer for 19% of businesses (Ecommerce News). In most of the tools that process customer data, CRM at the top of the list, multiple customer records for the same customer show as several different contacts. Being able to access all the information spread across several records becomes an impossible task. This results in incomplete customer knowledge, more mailings than necessary in campaigns, and that are also poorly targeted.
Ideally, to remedy this, it is best to qualify identification and contact data of target customers as soon as they are entered. When a company can send messages to known contacts that it recognizes and that it can reach, it can boost marketing campaign performance.
2. Putting a stop to using haphazard customer data to sell and innovate
Selling, steering, and innovating are key business development areas. Customer data that is of uncertain quality can be an obstacle to focusing on these areas.
First, lead quality is often lacking. According to Cognism, 40% of lead data in companies is inaccurate. Sales teams therefore have to make multiple adjustments and verifications to classify, contact, and qualify the customer profiles that are in the conversion tunnel. As such, no less than 68% of companies report that poor data quality has a negative impact on their sales pipeline (Harvard Business Review)! This problem was discovered at Terreal Wienerberger, as explained by Miguel Moreau, Digital Project Manager: “How can I see better data input today, and how can it be more complete? And most importantly, how am I going to convince and help (…) a sales colleague to not go too quickly when entering data, and to avoid entering incorrect data so that they can save time?” These multiple questions have a single solution: data quality.
Strategic decisions become haphazard if they are based on unreliable customer data and duplicate records. Bad data quality is a source of worry for 84% of CEOs (Forbes), given that up to one quarter of all data in large companies is inaccurate (Gartner). This fear on the part of CEOs is justified: it is difficult to count on KPIs when duplicate records give an inaccurate number of customers in the database, and so a unified view is lacking. This was an issue tackled by Carrefour, with digital contact with customers taking center stage for food retailers. “This change made us focus on the CRM asset first. (…) There is the subject of silos that has always been with us, (…) but getting a bird’s-eye view of the issues ends up not being so easy,” said Charles Giannesini, Loyalty, Strategy, and Customer Activation at Carrefour Hypermarché.
Innovation is also at risk when data quality is lacking. This is particularly important when we talk about AI. Without qualified data, AI results end up being questionable and present a considerable obstacle to deploying innovative use cases.
In the end, these development challenges all need to have a strong data foundation to speed up results and make them reliable. Tools for deduplicating and merging customer data take care of a large portion of the problematic inaccuracies.
3. Putting a stop to unnecessary treasury outflows
According to Gartner, 60% of companies do not estimate the financial toll that poorly qualified data takes on their business. This toll is still a very real aspect of operations.
Mail and packages that do not get delivered due to address errors are a considerable expense for many companies, primarily e-commerce retailers. According to MetaPack, for e-commerce alone, address errors are the cause of a 20% delivery failure rate. Each undelivered item means having to recontact the customer, verify their address, and resending the item. This logistical and financial hurdle comes from poorly input postal address data that is inadequately standardized, and as such it is difficult to use.
Another source of extra expense due to poor data quality is incorrect addressing and identification on invoices. The problem, once again from incorrect data input, can be more acute with B2B clients due to their complex identification data. If this data is incorrect, it can quickly create confusion between the entity being invoiced and the head office. And reissuing an invoice or drafting a credit note slows incoming cash.
These scenarios share a common trait: systems that use incorrect customer data and that have duplicate records – up to 20% of CRM system data consists of duplicate records, according to Experian. An ERP tool, just like a CRM tool, cannot deliver reliable results without qualified and unified data.
Ivelina Burden, Director of Organization and Processes, Rossel Advertising France, explained: “You have to consolidate data that comes from different sources (such as) websites, ERPs on different platforms (…). The main idea is to consolidate this data, update it, practically in real time, then make access to it super simple for the business and for all stakeholders.”
Having control over the quality of customer data streams in these systems starts as soon as the data is collected, with verification and autocomplete of input fields in real time, and continues in the database with curative data quality processing.
4. Not running the risk of regulatory sanctions
GDPR requires that companies rigorously manage their customer data. To that end, data quality provides support for meeting several GDPR requirements. An example is ensuring compliance with data retention requirements. For this, verified and complete contact data feeds the scoring of actual interactions with the customer in order to provide justification for the length of time their information is kept in the database.
For B2B, companies are gearing up for another major compliance requirement, e-invoicing, which is going to change the rules regarding administrative audits. Random audits are a thing of the past: invoice flows will now be continually automated. But company legal information changes quickly: as an example, 59 companies move to a new address every hour. As well, 33% of B2B data becomes obsolete each year (Dun & Bradstreet). “As companies do business, are bought out, and transform, they are not always thinking about their suppliers”, explained Sylvain Alunni, Head of Business and Project Director at SFR Business. This is why it is important “from the get-go to obtain customer data that is verified, controlled, and validated, but also to keep data that way throughout the customer relationship.” Focusing on data quality with B2B data helps keep an eye on changes, update company registration numbers, and standardize postal addresses in the database as well as in real time on input. This guarantees compliance with e-invoicing requirements.
5. Not tarnishing its reputation
For 49% of sales professionals, poor data quality negatively impacts company reputation, according to Forbes. Reputation is decided on two fronts:
Digital reputation: a cleansed customer database guarantees that you can reliably reach customers, key for reaching your target. In emailing campaigns, valid email addresses ensure a near 100% deliverability rate. With such reliable results, the company runs little risk of seeing its reputation diminished among internet access providers, and being blacklisted. From a customer and prospect point of view, targeted and personalized campaigns strengthen the brand image and increase the opening and conversion rates.
Compliance reputation: when companies have cleansed and reliable data, they can be counted on within their ecosystem. Pixmania’s marketplace is one example: “The financial partners we work with are very demanding in terms of data quality and the correctness of the information we give them,” explained Claire Menetrier, E-commerce Manager. This is quite a sensitive issue in banking and insurance, and in financial services, which include KYC and anti-fraud requirements.
In addition, the rapid pace of technological change creates new opportunities for fraud. This can include identity theft, SIM swapping, and falsifying supporting documents. The solution: taking advantage of mobile subscriber data via APIs through mobile telephone operators’ systems. This means that data quality can identify recent activations of SIM cards, as well as verifying that customer identification information corresponds to the information stored by the mobile telephone operator. This type of matching, alongside controls for data quality at input and deduplication, pushes managing the risk of poor data quality into high gear.
In the end, customer data flows into most company systems. This is why qualifying these flows makes these systems more relevant and contributes to their delivering results, and to a fair and reliable view of customers. This means that companies can therefore establish a foundation for good performance and relevance for facing strategic challenges.
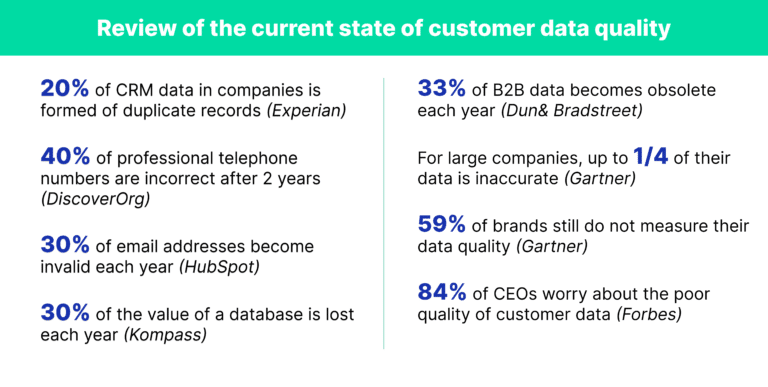
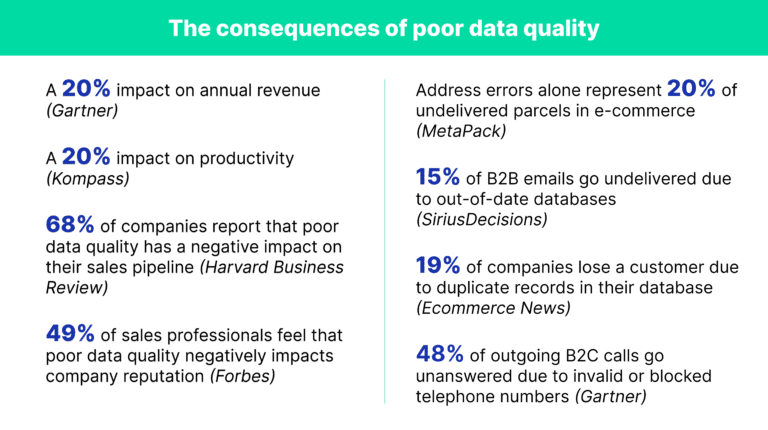
About DQE
Because data quality is essential to customer knowledge and the construction of a lasting relationship, since 2008, DQE has provided its clients with innovative and comprehensive solutions that facilitate the collection of reliable data.
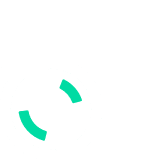
17
Years of
expertise
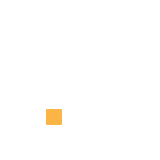
800
Clients in all
sectors
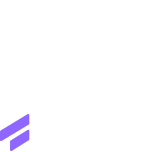
3Bn
Queries per
year
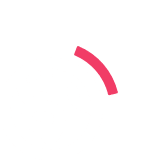