3 mistakes that jeopardize your data quality management
Data quality is the basic foundation of a data-driven company, offering functions that make both incoming data, and data within databases, reliable. Given the many processes and systems that use customer data, data quality requires real management to be applied at all levels. Here are 3 pitfalls to avoid in order to properly control data quality management.
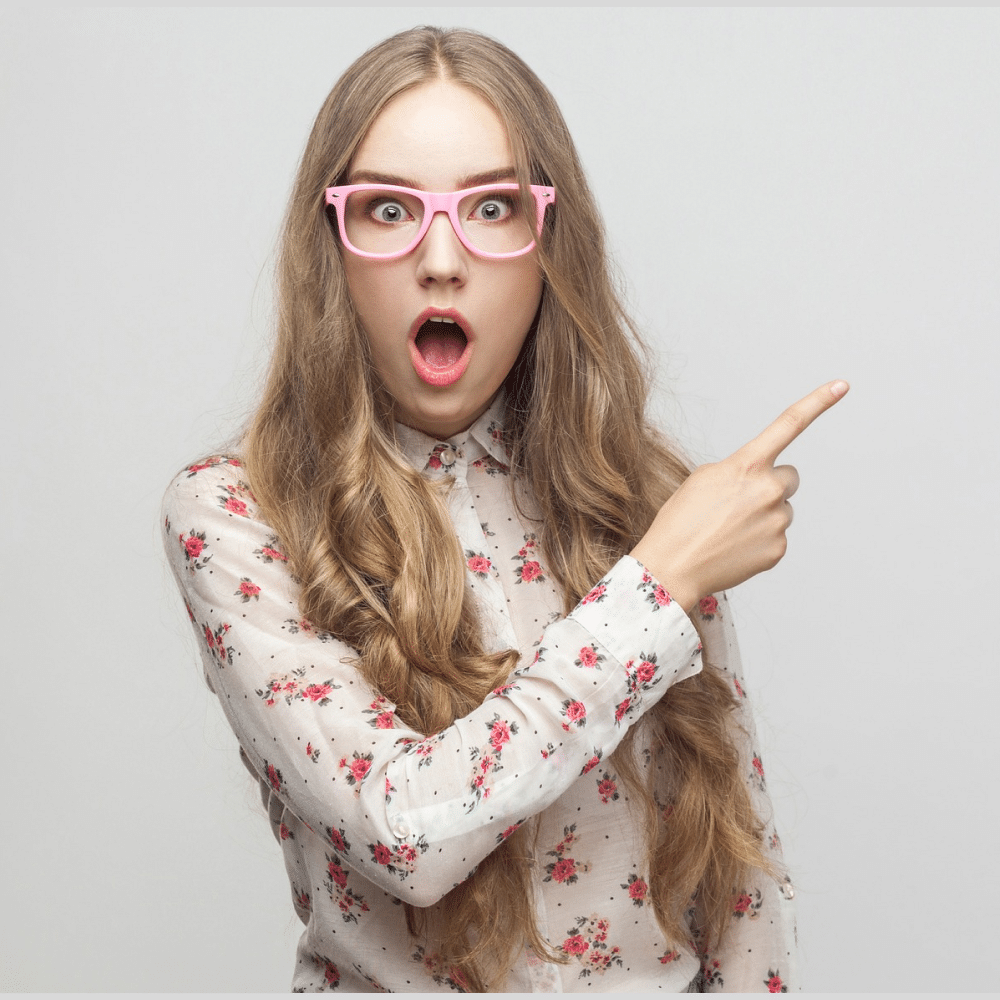
Whether it be CDP, CRM, UCR, or marketing automation, in any company, a project that involves customer data also involves data quality issues. If data quality is not controlled at the beginning of a project, it can be a source of problems and degrade the efficiency of the activities carried out by the users of the business line systems.
Strong Data Governance must include data quality management
Your users, tools and processes need quality data! Let’s take CRM as an example, as it is a tool used by a majority of companies. A CRM tool cannot function without data that is qualified and unified. When duplicate records form more than 10% of the records in a database, and 25% of the database’s contact emails are incorrect – proportions observed by our teams in companies that have not applied data quality functions and processes – the CRM tool cannot perform miracles, and ends up propagating poor quality data in your different systems. Consequently, users cannot take full advantage of the tool’s added value because they cannot trust the data they are using to properly serve the customer relationship.
However, a CRM is not designed with strong data quality management functions in mind. According to companies that produce CRM tools, some customer relationship tools do include certain data quality functions. These are often very limited. Deduplication is an example: a CRM tool can identify duplicates only if they are perfectly accurate on a single criterion – usually the customer’s name or email address. Far from being able to handle all types of duplicates, CRM tools also lack the processing power required to unify very large databases of several tens of millions of customers. The only thing left to do is to work with data quality to get the most out of the CRM tool.
The problem is universal since it concerns all the systems that use customer data. However, the success of a customer data quality management approach can be hampered by 3 errors:
1. “My customer data is of good quality, there is no need for it to be controlled and cleaned”
In any business, customer contact information is exposed to too many process flaws, themselves generating erroneous data. Human errors in data entry, changing phone numbers or addresses, importing databases that are of poor quality… All databases are subject to the problems that come from recording bad data.
In terms of duplicate records, their share in databases averages between 5 and 10%. At 10% and above, a critical threshold is reached where the effectiveness of customer contact and marketing programs and decision-making is degraded. The main source of duplication comes from the compartmentalization of the company’s various information systems, which multiply the databases involved, such as the CRM marketing/sales database, customer service, ERPs, points of sale, or the web portal. At each touchpoint, the collection of customer data is likely to trigger the creation of a new customer file. Inexorably, duplicates spread throughout the company. However, each department is aware of the value of quality information.
But these previous examples are not the only sources. Duplicates can also come from certain organizational biases, especially when the creation of new customer records is incentivized from a new business perspective. This practice encourages operational staff to systematically create a duplicate customer record, even if it means using workarounds when an operational tool blocks multiple entries of the same email address. A small change, such as an extra dot in an email address, or a deliberate inversion of letters, can “force” the system to identify the record as distinct.
2. "We develop our own data quality management solution": THE thing to avoid!
Our experience with several companies confirms that those who have tried to deduplicate and merge their customer data on their own have lost huge amounts of development time and money, with results that fall far short of the requirements in the domain.
It is not uncommon to see processing times of several weeks with in-house solutions, when a specialist solution could do the work in a few hours. Moreover, the identification of duplicates leaves much to be desired when a solution is too generic, or when it does not use reconciliation keys that are granular enough to find a maximum number of duplicates in a database. Finally, there is the case of companies that stop the process when it comes time to merge records due to lack of control and fear of losing customer data.
3. “Let’s give the company’s DQM to a project-specific integrator”: not such a simple task...
Entrusting a data quality project to non-specialists exposes your company to several possible setbacks. One of the reasons is that a non-specialist does not have a vision of all the needs associated with data quality, nor the hindsight on the technical issues, processing times, and pitfalls to avoid at the POC stage. In order to successfully complete a data quality project, the best strategy is to use a specialist solution that has already been developed and tested, that is immediately operational.
Advice and support are also essential in order to take advantage of best practices and proven benchmarks in the success of a data quality project. So, when it comes to deduplication, we need to know where to stop, and to understand that the goal of identifying 100% of duplicates is not pragmatic. Without the guidance of a specialist, this best practice is unlikely to be applied, yet it contributes to the successful integration of data quality with objectives that are realistic and that are achieved.
About DQE
Because data quality is essential to customer knowledge and the construction of a lasting relationship, since 2008, DQE has provided its clients with innovative and comprehensive solutions that facilitate the collection of reliable data.
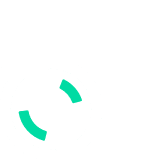
+15
Year of
expertise
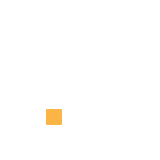
+400
Clients in all
sectors
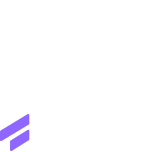
3Md
Queries per
year
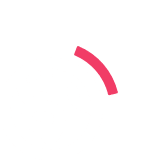
+240
Internationnal
repositories
Our latest resources
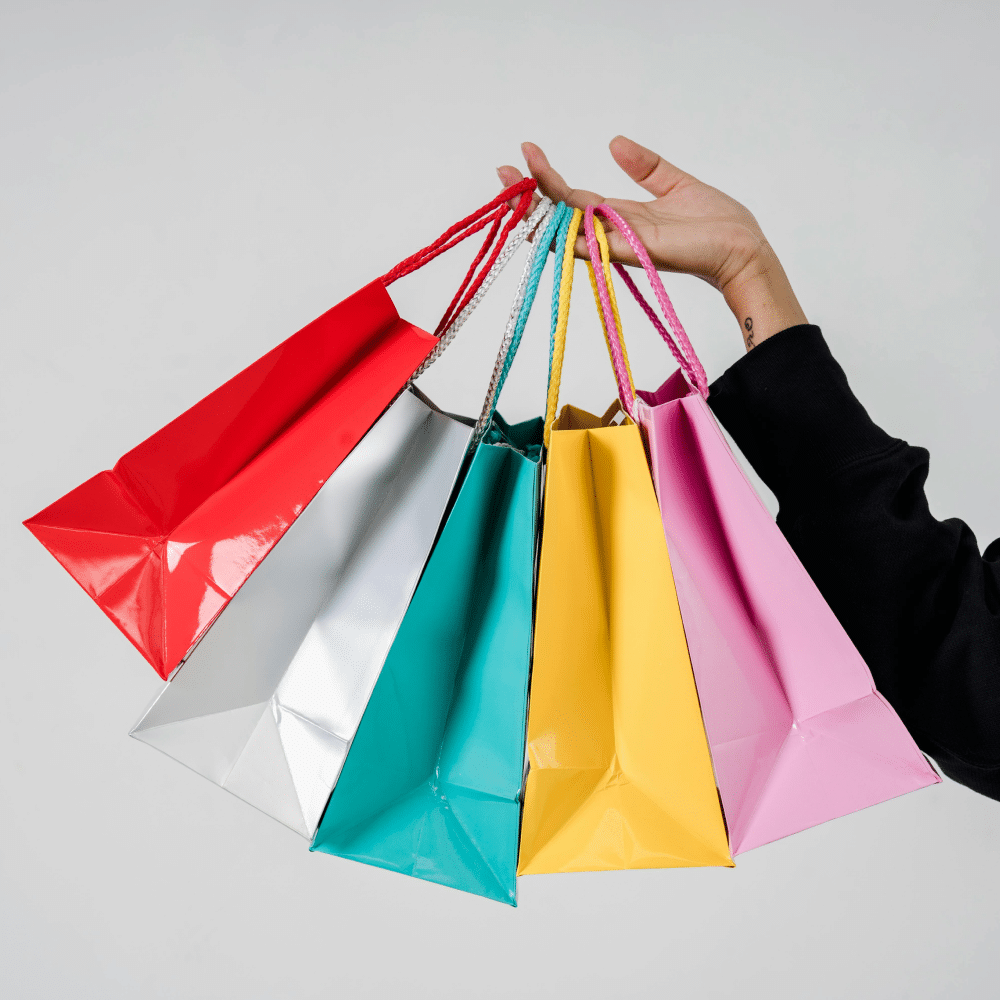
April 19, 2024
Retail: Are you activating data quality management in your customer loyalty strategy?
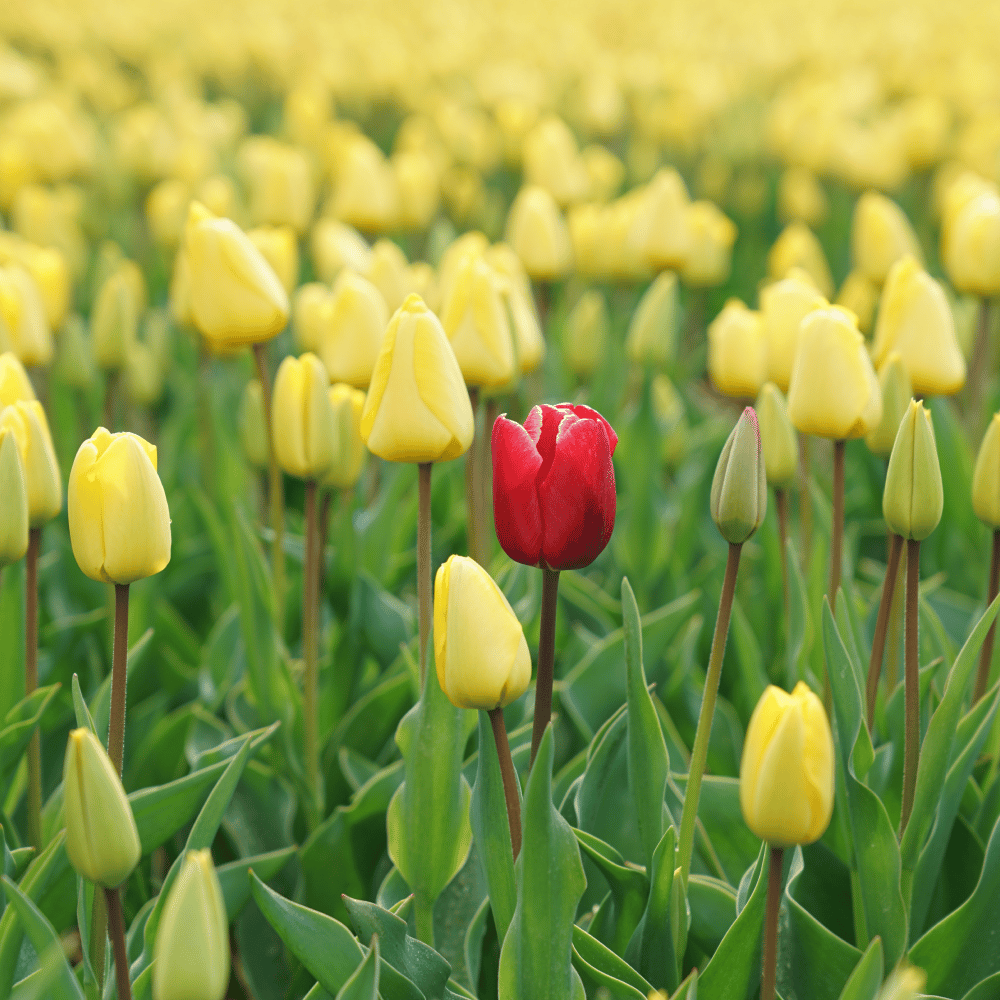